The Challenge
Lundy is a real estate search engine designed for MLS (Multiple Listing Service) organizations. Their aim is to make real estate data more accessible and inclusive. One of their core MLS tools is the Navigator AI app which Transitiv helped build. Navigator is a knowledge assistant built using RAG (Retrieval-Augmented Generation) to solve a critical problem that MLSs face – finding the correct information.
MLS organizations handle a vast amount of internal documentation — from compliance guidelines to operational policies and training manuals. Finding specific information within these documents is a time-consuming task. Lundy wanted to develop a solution that helps solve this. Transitiv’s AI integration services came to the rescue.
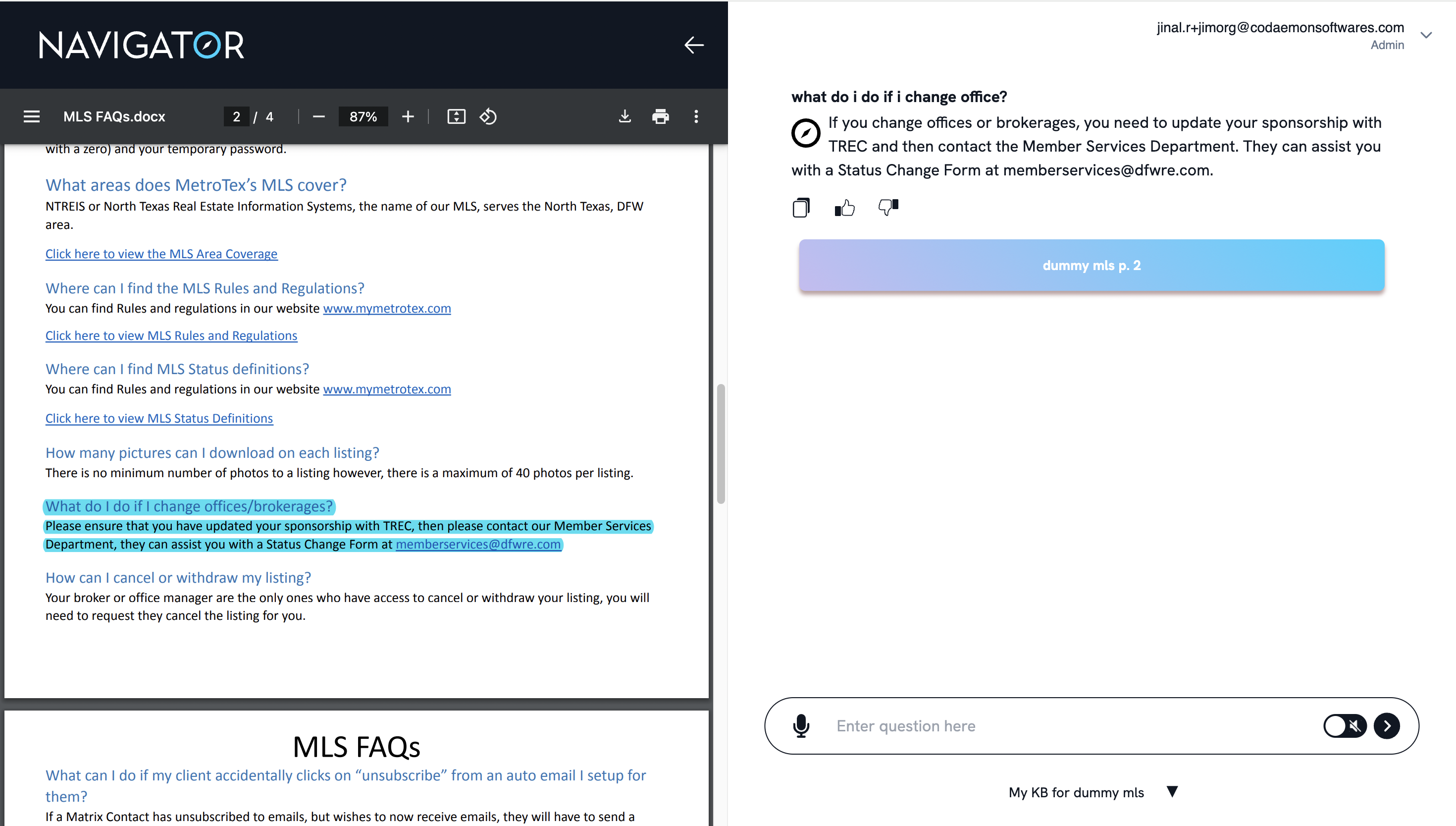
Solution
Transitiv worked with Lundy to build the core of their Navigator AI app using RAG. This AI integration is designed to make information retrieval easier and quicker. But before we delve into the details of the app, let’s simplify what an RAG is.
Retrieval-Augmented Generation is a technique that enables Large Language Models (LLMs) to reference a trusted, external knowledge source to generate answers. Much like how a personal assistant looks up your schedule to suggest a meeting time. This makes the LLM output accurate and highly relevant.
Now let’s take a look at how the RAG solution for the Navigator app works:
1. Data Ingestion: This is where the external knowledge source comes in. An MLS can upload its knowledge base to the tool in 2 ways:
- Manual upload: As individual files or in bulk; in PDF, HTML, Text format
- Automatic upload: We implemented a web crawler that could be scheduled to automatically pull content from the MLS’s internal knowledge base website.
2. Document Processing:
Once files are uploaded, the system processes it into text chunks and stores them along with their context (such as where the content appears in the document). Each chunk is then converted into embeddings and stored in a vector database. What this does is, it turns the dense manual into a filing system of flash cards which can be pulled out when a user asks a question.
3. Information Retrieval & Annotation
The core functionality of Navigator is its ability to provide accurate, quick answers from large sets of internal documents. When someone types a question in the tool, the system performs a vector search to find the most relevant information. It comes back with a direct answer and a link to the exact document section from where the information was taken.
To keep the knowledge base up-to-date, the crawler can be scheduled to scan the source website regularly, ensuring the latest content is always available. The tool also supports voice queries and output enabling hands-free interaction.
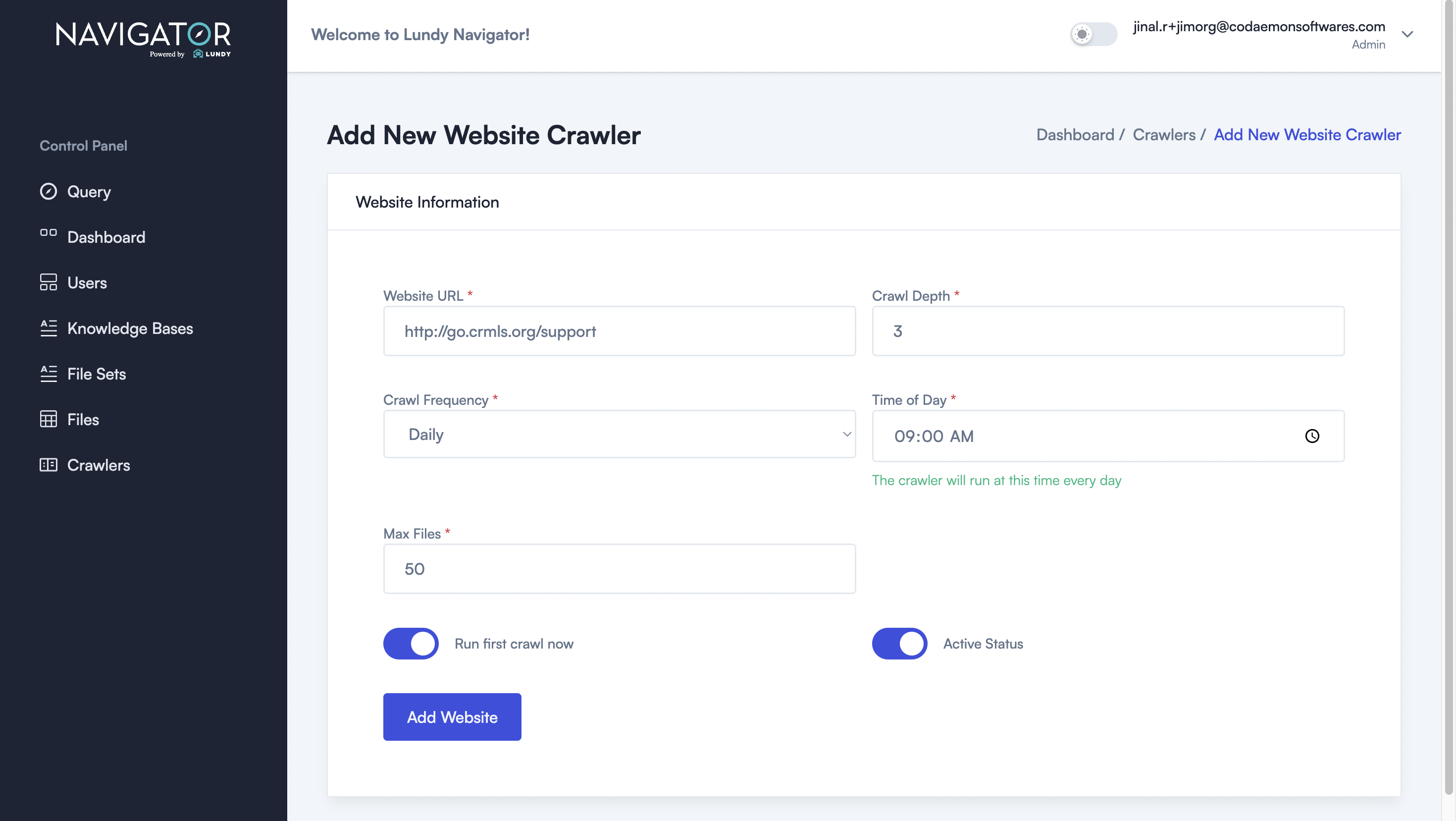
Key Outcomes
Our AI integration work with Lundy transforms the way MLSs access information and achieves the following:
- Reduced response time: Eliminates the need to search through documents manually.
- Reduced workload: No more hours spent addressing repetitive questions; Navigator handles it automatically.
- Accurate answers: Annotation for every answer ensures clarity and correctness.
- Updated knowledge base: Automatic crawling keeps information current.
While originally built for real estate, this engine is versatile and can benefit any industry that deals with volumes of internal documentation.